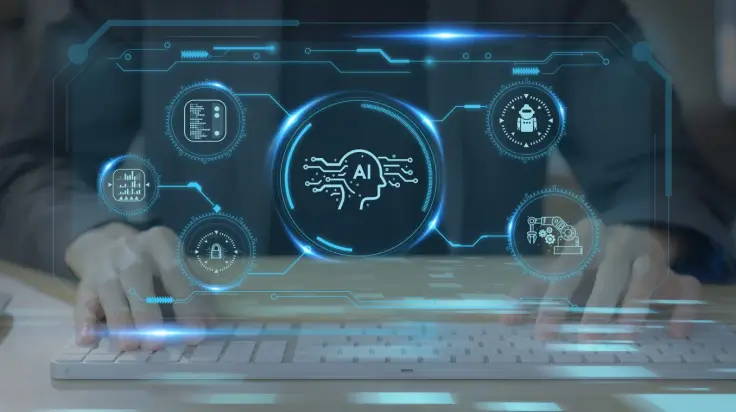
In recent years, the field of AI has shown impressive progress and innovation, affecting our everyday lives in unprecedented ways. One of the very recent tools available to us, which has changed the way we work, is “Chat GPT”. This tool has created a lot of commotion in the way we operate every day. The model it operates on is called a Generative model. One needs to understand the basic difference between the now-normal concept of Machine Learning and the Generative model.
Generative AI and machine learning are related fields within artificial intelligence, but they serve different purposes and have distinct characteristics. Let’s explore the differences between them:
Purpose:
Machine Learning (ML): Machine learning is a broader field that encompasses a variety of techniques for training models to perform specific tasks without being explicitly programmed. ML can be used for tasks such as classification, regression, clustering, and more. It is often used for predictive modelling and decision-making.
Generative AI: Generative AI focuses on creating data, typically in the form of images, text, audio, or other media. It aims to generate new content that resembles human-created data. Generative AI models are used for tasks like image generation, text generation, and artistic creation.
Training:
Machine Learning (ML): In ML, models are typically trained on labelled datasets to learn patterns and make predictions or classifications. It involves supervised learning, unsupervised learning, and reinforcement learning.
Generative AI: Generative AI models are trained using unsupervised learning techniques. They learn to generate data by observing and capturing the patterns and statistics of the training data. For example, a generative adversarial network (GAN) is a common framework used in generative AI.
Output:
Machine Learning (ML): ML models typically output predictions or classifications based on input data. For example, a spam filter classifies emails as spam or not based on their content.
Generative AI: Generative AI models produce new data samples that are not present in the training dataset. For instance, a text-based generative AI might produce human-like paragraphs of text, or an image-based generative AI can create images of imaginary animals.
Applications:
Machine Learning (ML): ML is widely used in various domains, including natural language processing, computer vision, healthcare, finance, and more. Applications include recommendation systems, image recognition, and speech recognition.
Generative AI: Generative AI is commonly used in creative applications, such as generating art, music, or fictional text. It is also used in data augmentation, style transfer, and creating realistic deepfake content.
Examples:
Machine Learning (ML): Examples of ML techniques include linear regression, decision trees, random forests, support vector machines, and deep learning with neural networks.
Generative AI: Examples of generative AI models include GANs (Generative Adversarial Networks), VAEs (Variational Autoencoders), and Transformers used in text generation, like GPT-3.
In summary, while both generative AI and machine learning are subsets of artificial intelligence, they have different focuses and applications. Machine learning is primarily used for predictive and classification tasks, whereas generative AI is used for creating new data and content that mimics human-generated data.
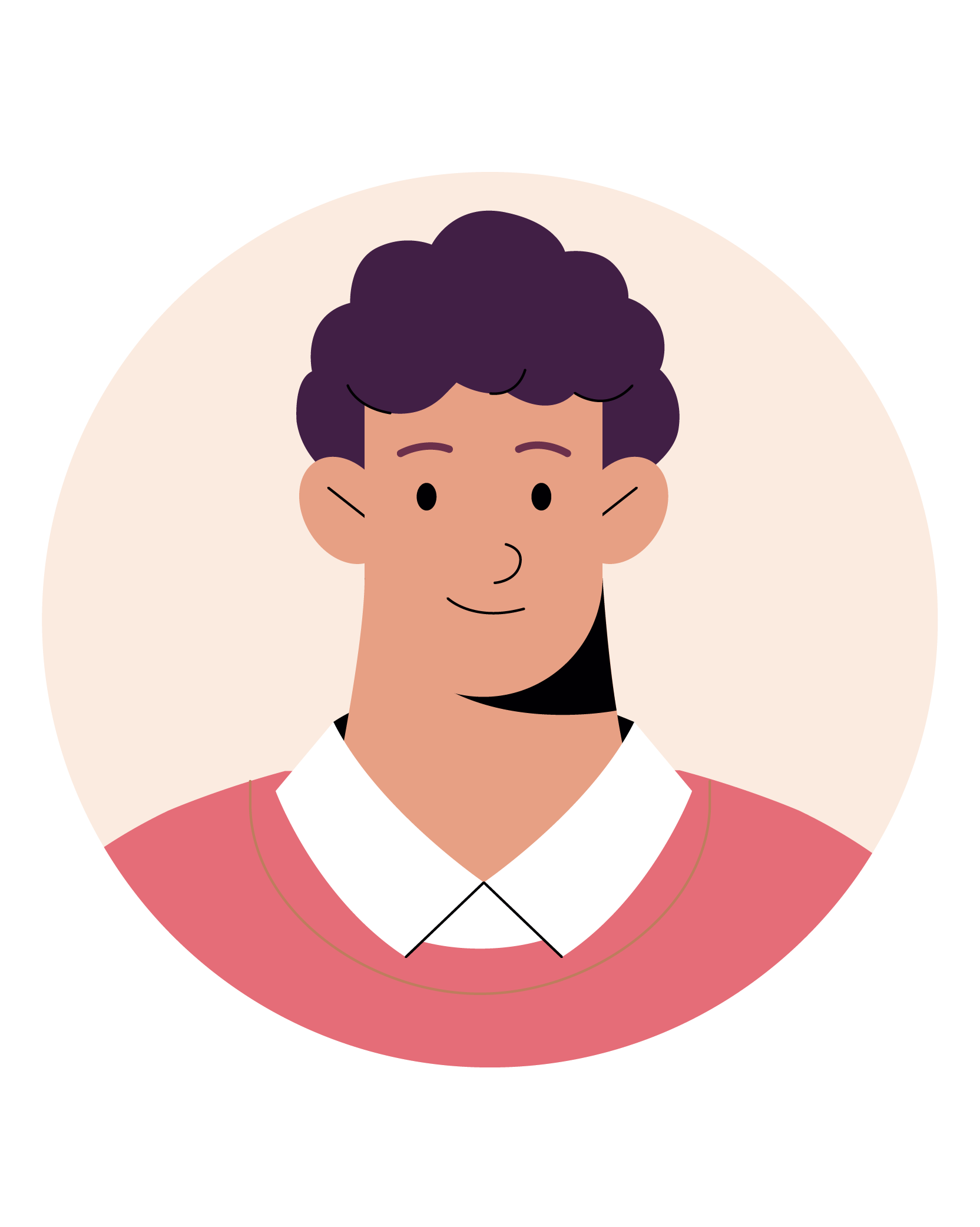
Dr. Kingshuk Srivastava
The writer is Dr. Kingshuk Srivastava, UPES School of Computer Science
UPES Admission Enquiry
Trending Post
Related Articles
Subscribe to UPES Blogs
Join our community for exclusive stories, insights, and updates
By clicking the "Subscribe" button, I agree and accept the privacy policy of UPES.