Transforming Data into Knowledge: The Role of Machine Learning in Computer Science
- Dr. Priya Ranjan
- Published 22/09/2023
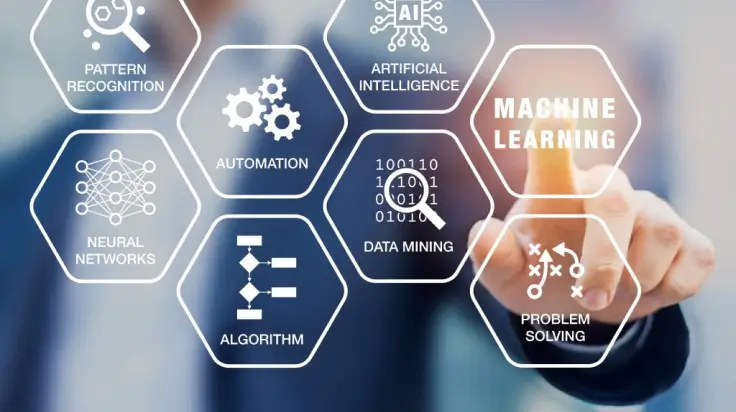
An exploration of the geometric study of computational disease tagging of ECGs has been presented to highlight the steps involved in transforming data into knowledge. Using computational perceptual similarity metrics like Earth Mover’s Distance (EMD), an automated clustering of healthy and unhealthy ECGs is demonstrated. This work illustrates that not only one can generate useful knowledge from seemingly innocent ECG data, but also use this knowledge to save lives in areas where cardiologists have not reached. When coupled with web-service-like interfaces, it can provide solutions to multiple cardiovascular diseases (CVD) in Low and Middle Income Countries (LMICs) like India. We suggest further ways to augment and enhance this automated classification scheme using bio-markers like Troponin isoforms, CKMB, and BNP using computational data fusion algorithms. Future directions include the study of larger datasets of ECGs from diverse populations collected from a heterogeneous mix of patients with different CVD conditions. Further, we advocate the robustness and stability of this programmatic approach as compared to deep learning kind of disease tagging schemes which are amenable to dynamic instabilities. Such instabilities are not acceptable in automated clinical digital trace processing systems.
Cardiovascular diseases (CVD) result in 17.9 million (31%) deaths each year worldwide. More than 75% of CVD deaths occur in Low and Middle Income Countries (LMICs). India has seen a significant rise in CVD-associated mortality rates with an epidemiological transition to non-communicable diseases. This pattern is uniform throughout the country despite wide variation in risk factors and socioeconomic status. India faces a great challenge in providing quality healthcare, especially in rural domains due to a lack of resources and trained healthcare providers. The lack of resources for triaging or stratification of patients based on the severity of their condition leads to prolonged waiting periods for treatment, further worsening the prognosis of the patients. Moreover, the scarcity of specialised cardiologists significantly impacts the clinical prognosis of the patients intensifying the cardiovascular disease burden.
The electrocardiogram (ECG) is a fundamental tool in the everyday practice of clinical medicine, with more than 300 million ECGs obtained annually worldwide. The ECG is pivotal for diagnosing a wide spectrum of cardiovascular abnormalities ranging from Arrhythmias to Myocardial infarction (MI). The hospital-based registries have neither been able to provide accurate estimates of the cardiovascular disease (CVD) burden nor identify the disease drivers for the CVD epidemic despite it being the largest cause of mortality. This necessitates the need to develop novel bottom-up strategies to map out the burden of CVDs at the community level as well. Such a strategy would not only help us to elucidate the niche-specific disease drivers but also augment the hospital-based registries in visualising the realistic burden of CVDs across the Indian sub-continent.
Although a large number of drugs have been designed for treating patients afflicted with CVDs across the Indian subcontinent, the absence of a systematic database taking into account the vast genetic base of the Indian populace has been perhaps the Achilles heel in developing policies or programs aimed for better management of CVDs. Development of novel Artificial Intelligence (AI) enabled Electrocardiogram (ECG) interpretation has become increasingly important in the clinical ECG workflow since its inception over 50 years ago, serving as a crucial adjunct to physician interpretation not only in resource limited clinical settings prevalent across the Indian sub-continent but also elsewhere across the world. The availability of affordable, accessible, and scalable computational platforms with capabilities to process large-scale raw data will not only improve expert human ECG interpretation by accurately triaging or prioritising the most urgent conditions but also importantly reduce the rates of misdiagnosed ECG interpretations. To this end, we are proposing a novel R-based open source software with the inherent capability to classify different kinds of automated geometric visualisations of ECGs along with its categorisation based upon similarity indices as measured by Earth Mover’s Distance (EMD).
We anticipate that integration of this robust automated classifier along with minimally invasive detection of molecular biomarkers such as N-terminal prohormone of brain natriuretic peptide (NTpro-BNP), Creatinine Phospho Kinase Muscle- Brain (CPKM/B), and Troponin isoforms will form the rationale for development of effective and precision oriented triage system for achieving not only high screening rates for Myocardial Infarction (MI) but also accurately triaging or prioritising the most urgent conditions. Biomarkers are emerging as a new technique to find precursors of many diseases including Epilepsy.
This work shows that using basic geometric ideas like Euclidean distance or similarity detection metrics like Earth Mover’s Distance used frequently in face detection problems can provide up to eighty percent accuracy in healthy ECG tagging. This can help the PHCs to make sense of ECGs using this work and further provide referral advice to patients. Integration of visual imaging-based tools with biomarkers like Troponin isoforms, CKMB, and BNP may enhance the capability to automatically categorise ECGs correctly but also may add to the instrumentation and costs. Future software will be expected to classify given ECG in these five categories with some confidence using similarity-based algorithms like EMD has many different implementations based on what kind of distancing we pick, i.e. “Manhattan” distancing is likely to give a different result from the emdL1 scheme.
We can even deploy drastically different methods like Visibility graph (VG) where different aspects of time series data can be used to automatically label healthy and diseased ECGs. Both EMD and VG can even give rise to a hybrid scheme if performance is boosted! A major future direction is to study the interspersed nature of healthy and unhealthy ECG vectors in clustering space to provide guidelines for disease tagging when it is too close to call, or it is obfuscating. Further indecision points can arise where EMD from both healthy and unhealthy ECGs is equal up to the second place of decimal. This ECG is equidistant from the healthy and unhealthy world in equal magnitude. Such problems arise because of the limited size and diversity of datasets and there is a natural need for larger and more heterogeneous datasets of ECGs apart from other modalities like Cardiac Auscultation and bio-markers.
Finally, one of the major questions with which the Indian clinical community has been struggling is which ECG or any other clinical digital trace e.g. cervigram, mammogram, etc. should be tagged “Healthy” when there is so much variation in what clinicians mean by a “Healthy” ECG. This work successfully picks a representative “Healthy” ECG from an ensemble of “Healthy” ECGs using Earth Mover’s Distance (EMD) based on the premise that this representative “Healthy” ECG should demonstrate maximum similarity to all other “Healthy” ECGs in a given ensemble. It is interesting to note that a serious issue of the medical community finally gets resolved in the world of computational similarity and machine learning.
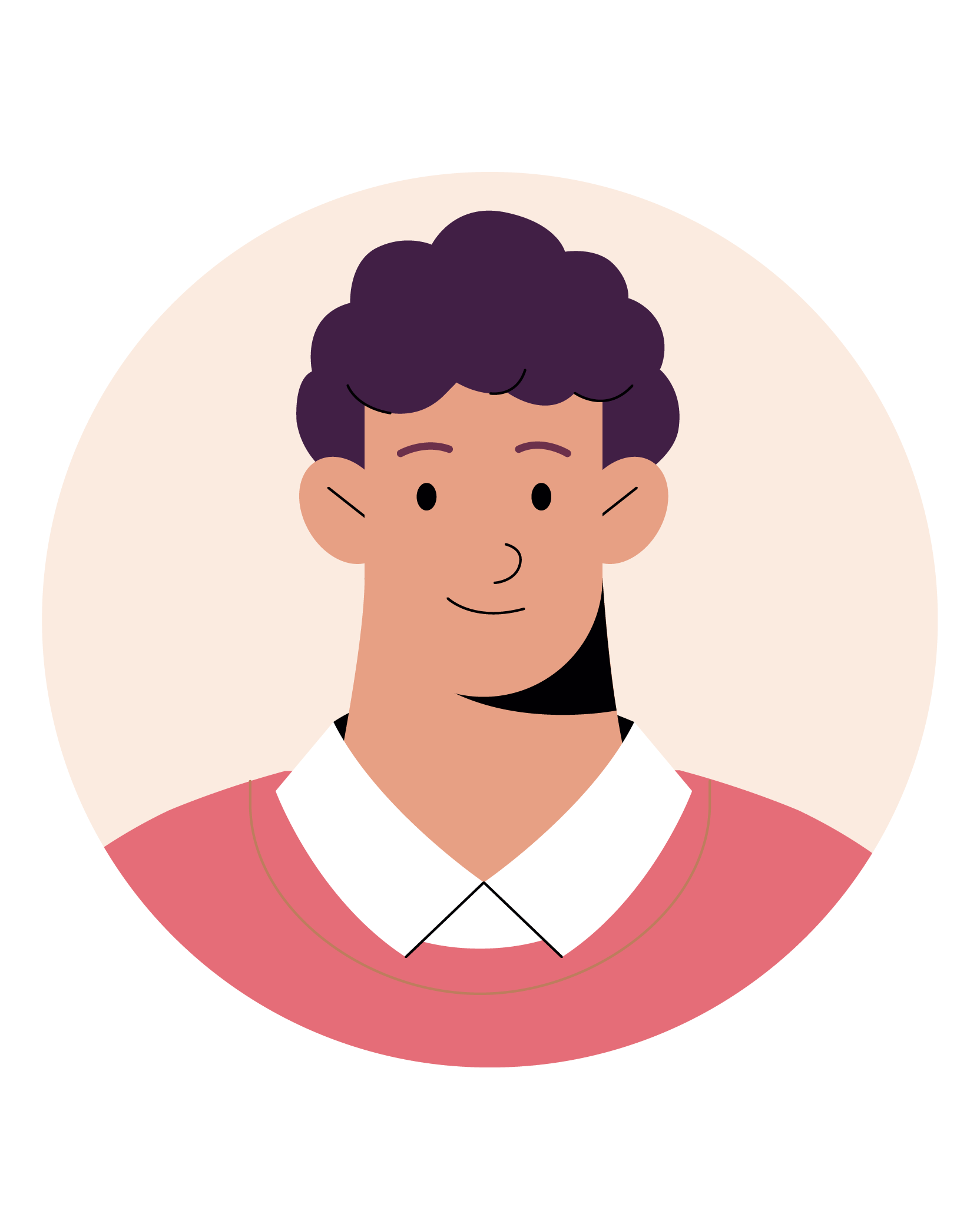
Dr. Priya Ranjan
The writer is Dr. Priya Ranjan, Professor, UPES School of Computer Science
UPES Admission Enquiry
Subscribe to UPES Blogs
Join our community for exclusive stories, insights, and updates
By clicking the "Subscribe" button, I agree and accept the privacy policy of UPES.